Knowledge Governance: The Essential Guide
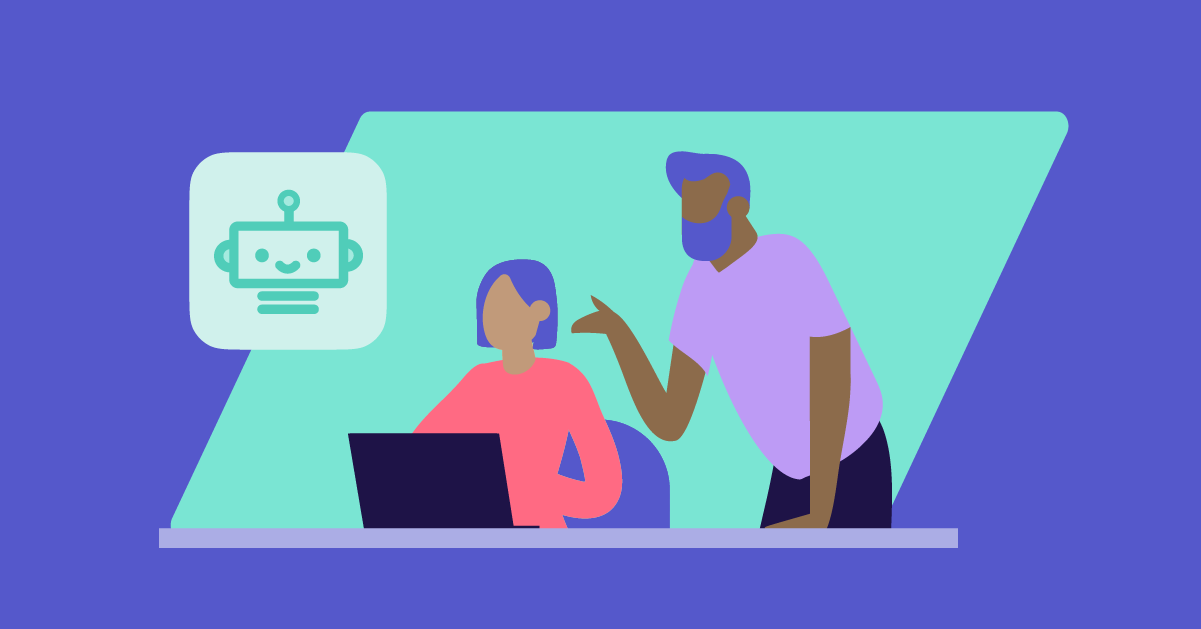
The effectiveness of automation and AI heavily depends on the quality of the knowledge they are built upon as 100% of generative AI projects that lack integration to modern knowledge management systems will fail to meet their customer experience and operational cost-reduction goals.
Knowledge governance, the framework for managing an organization's knowledge assets, is central to ensuring that AI implementations are successful. This blog will explore how knowledge governance plays a pivotal role in AI success, its importance, components, benefits, and strategies for ensuring AI systems are as good as the knowledge they leverage.
What is knowledge governance?
Knowledge governance refers to the systematic management of an organization's knowledge assets, encompassing the policies, processes, roles, and technologies that ensure knowledge is effectively captured, shared, and utilized. It acts as a framework to guide how knowledge is created, maintained, and applied within an organization, especially when deploying AI systems.
Effective knowledge governance ensures that the knowledge feeding AI systems is accurate, consistent, and aligned with organizational goals and regulatory standards. It involves establishing clear guidelines on data quality, access control, privacy, and security to ensure that AI systems operate on reliable information. Without robust knowledge governance, organizations risk basing AI decisions on poor-quality data, which can lead to faulty insights and compliance risks.
Why do you need knowledge governance before implementing AI?
Understanding the importance of knowledge governance in AI is crucial for organizations looking to leverage AI technologies. Effective knowledge governance ensures that AI systems operate with high-quality data, align with business objectives, and comply with regulations. Without proper governance, AI systems can produce unreliable results, leading to potential risks and missed opportunities.
Alignment with business objectives
Knowledge governance ensures that the knowledge used by AI systems aligns with the organization's strategic goals. This alignment maximizes the value derived from AI implementations, ensuring that AI-driven decisions support overall business objectives.
Data quality and integrity
AI systems require high-quality, well-governed data to function correctly. With 77% of the average US company’s data being redundant, obsolete, trivial, or dark data, knowledge governance ensures that data is accurate, consistent, and free from biases that could compromise AI outcomes, thereby enhancing the reliability of AI insights.
Regulatory compliance
Many industries have strict regulations regarding data handling. Knowledge governance helps organizations ensure that their AI systems comply with these regulations, avoiding penalties and ensuring ethical AI use by adhering to legal standards.
Risk management
Proper knowledge governance minimizes risks associated with data breaches, biased AI outputs, and misuse of information. It ensures that AI systems are secure and reliable, reducing potential threats and vulnerabilities.
Improved AI decision-making
Structured knowledge governance ensures that AI systems have access to accurate and relevant information, leading to better decision-making and more reliable AI predictions. This results in higher confidence in AI-driven processes.
Knowledge governance challenges to overcome
The principle of garbage in, garbage out is critical—poor-quality inputs lead to unreliable outputs. If an organization lacks proper controls over how knowledge is created, categorized, and maintained, the resulting decisions and processes will be flawed. Without solid knowledge governance, AI and automation will increase inconsistencies, inefficiencies, knowledge gaps, and compliance risks.
Strong governance ensures that only accurate, relevant, and well-structured knowledge enters the system, enabling reliable decision-making and operational excellence. Without it, even the most advanced knowledge management tools will fail to deliver value.
Knowledge lives in silos and multiple systems
In many organizations, knowledge is spread across various systems and departments, creating silos that hinder access to comprehensive data. This fragmentation poses a significant challenge for AI systems, which require a unified and consistent knowledge base to function effectively. Without breaking down these silos, AI systems may produce incomplete or skewed results.
Expert knowledge is not being captured
Another critical issue is the failure to capture expert knowledge within the organization. Valuable insights and expertise can often be lost or remain in the minds of experienced employees, leading to knowledge gaps when they leave or change roles. This lack of documented knowledge can impede the development and accuracy of AI systems, which rely on comprehensive and detailed information to learn and make decisions.
Knowledge is outdated and unreliable
AI systems depend on current and accurate data to make informed decisions. However, many organizations struggle with outdated and unreliable knowledge as 45% of IT leaders reported that poor data management makes them unable to leverage emerging tech like generative AI. Regularly updating and verifying knowledge ensures that AI systems operate on trustworthy information, reducing the risk of flawed insights.
No ownership or accountability
A common pitfall in knowledge management is the absence of clear ownership and accountability for knowledge assets. Without designated roles to oversee knowledge governance, data can become neglected, leading to inconsistencies and gaps. Establishing accountability ensures that knowledge is maintained, accurate, and accessible, supporting AI systems in delivering reliable results.
Components of knowledge governance for effective knowledge management
Knowledge governance comprises several key components that work together to ensure the effective management of knowledge assets. These components include establishing policies and standards, defining roles and responsibilities, implementing knowledge management processes, leveraging appropriate technology and tools, and setting metrics for measurement. Each component plays a vital role in supporting AI systems with reliable and well-governed knowledge.
Knowledge policies and standards
These define the rules and guidelines for managing knowledge that feeds into AI systems. They cover areas such as data quality, access control, and data privacy, ensuring that the knowledge base remains robust and secure.
Roles and responsibilities
Clear roles and responsibilities are essential for effective knowledge governance. Key roles may include data stewards, knowledge managers, and AI ethics officers, each ensuring that knowledge is managed according to governance policies.
Knowledge management processes
These are the activities involved in capturing, storing, sharing, and utilizing knowledge for AI. Processes should be well-defined and integrated into the organization's workflows to ensure seamless AI operation and knowledge flow.
Technology and tools
The right technology is crucial for supporting knowledge governance. This includes knowledge management systems, AI training platforms, and analytics tools that ensure data is properly governed, accessible, and usable by AI systems.
Metrics and measurement
Establishing metrics to measure the effectiveness of knowledge governance in AI is essential. Common metrics include data quality scores, AI accuracy rates, and compliance rates, helping organizations track and improve their governance practices.
Top benefits of knowledge governance for business
There are many benefits of knowledge governance, ranging from increased AI accuracy to better compliance and risk management. These benefits ultimately lead to more reliable and effective AI implementations.
Ensures consistency and accuracy in processes
Knowledge governance ensures that AI systems operate on consistent and accurate data, leading to more reliable outcomes. By maintaining standardized processes for data management, organizations can reduce errors and inconsistencies in AI-driven processes.
By ensuring that only high-quality, governed knowledge feeds into AI systems, organizations can improve the accuracy and reliability of AI outputs. This leads to more precise and dependable AI-driven decisions.
Facilitates better decision-making and boosts confidence
With well-governed knowledge, AI systems can provide more accurate insights, enabling better decision-making. This enhances confidence in AI outputs, ensuring that stakeholders trust the decisions and actions derived from AI analysis.
Reduces risk of non-compliance
Poor data quality costs organizations an average of $12.9 million annually. A robust knowledge governance framework helps organizations stay compliant with industry regulations and standards. By managing data privacy and security effectively, companies can mitigate risks associated with non-compliance, avoiding legal and financial penalties.
Knowledge governance ensures that AI systems operate within legal and regulatory boundaries, reducing the risk of non-compliance and ethical breaches. This fosters trust in AI systems and their outputs.
Enhances collaboration and knowledge sharing
Knowledge governance promotes collaboration by breaking down silos and encouraging knowledge sharing across departments. This ensures that AI systems have access to a diverse and comprehensive knowledge base, enhancing their learning and decision-making capabilities.
A governance framework encourages knowledge sharing across departments, ensuring that AI systems have access to diverse and comprehensive data. This diversity enriches AI learning and decision-making processes.
Creates a foundation that scales
A well-structured knowledge governance framework provides a scalable foundation for AI systems. As organizations grow and their data needs expand, a scalable governance structure ensures that AI systems can continue to operate efficiently, supporting long-term business goals.
Strategies for implementing knowledge governance
Implementing knowledge governance requires a strategic approach. Organizations need to define clear objectives, develop a comprehensive governance framework, engage stakeholders, leverage appropriate technology, provide training and support, and continuously monitor and review governance practices. These strategies help ensure that knowledge governance is effectively integrated into AI initiatives.
Define clear objectives
Start by defining what you want to achieve with knowledge governance in the context of AI. Objectives should be aligned with business goals and address specific challenges the organization faces with AI, providing a clear direction.
Develop a governance framework
Create a comprehensive framework that includes policies, processes, roles, and metrics specifically for AI systems. This framework should be tailored to the organization's needs and AI strategies, ensuring relevance and effectiveness.
Engage stakeholders
Involve key stakeholders from different departments to ensure buy-in and support for AI-related knowledge governance. Stakeholder engagement is crucial for successful AI implementations, fostering collaboration and commitment.
Leverage technology
Implement the right technology to support knowledge governance for AI. This includes data management systems, AI development tools, and analytics platforms that ensure data quality and governance, enhancing AI's operational efficiency.
Provide training and support
Ensure that employees understand the importance of knowledge governance in AI and are trained in governance policies and processes. Ongoing support and resources should be provided to help them adhere to governance standards, ensuring consistent application.
Monitor and review
Continuously monitor the effectiveness of knowledge governance in AI and make necessary adjustments. Regular reviews will help identify areas for improvement and ensure that the governance framework remains relevant to AI advancements and organizational changes.
Future trends in knowledge governance and AI
Integration with AI and machine learning
AI and machine learning are transforming knowledge governance by automating processes, improving knowledge discovery, and providing insights for better AI decision-making.
Focus on data privacy and security
With increasing concerns about data privacy and security, knowledge governance frameworks will need to incorporate robust measures to protect sensitive information feeding into AI systems.
Enhanced collaboration tools
The rise of remote work and global teams is driving the need for advanced collaboration tools that support knowledge sharing and governance across geographies, ensuring AI systems have comprehensive data access.
Continuous improvement and innovation
Organizations will need to adopt a continuous improvement mindset, regularly updating their knowledge governance frameworks to adapt to changing business needs and technological advancements in AI.
Getting started with knowledge governance in your organization
Knowledge governance is a critical component of successful AI implementation, ensuring that knowledge assets are managed effectively to drive AI success. By implementing a robust knowledge governance framework, organizations can enhance AI accuracy, ensure compliance, foster innovation, and gain a competitive advantage. As AI continues to evolve, the importance of knowledge governance will only grow, making it an essential focus for organizations aiming to leverage AI effectively.
Visit our solutions page to learn more about how you can ensure your organization’s knowledge is ready for AI by creating a foundation of trusted knowledge that sets your team and AI up for success.